Benchmarking Energy Expenditure Estimation: AI vs. Metabolic Cost Models
In clinical and sports science applications, the accurate estimation of energy expenditure (EE) is critical for evaluating physical performance, rehabilitation progress, and overall health. Traditionally, EE has been estimated through metabolic cost models based on joint kinematics and musculoskeletal dynamics.
However, recent advances in AI particularly in machine learning and deep learning have opened new possibilities for estimating EE more directly from wearable sensor data, such as inertial measurement units (IMUs) and heart rate monitors. These data-driven approaches present an exciting opportunity for real-time, real-world application.
Building on this progress, this thesis aims to benchmark and evaluate different methods for estimating energy expenditure from movement data.
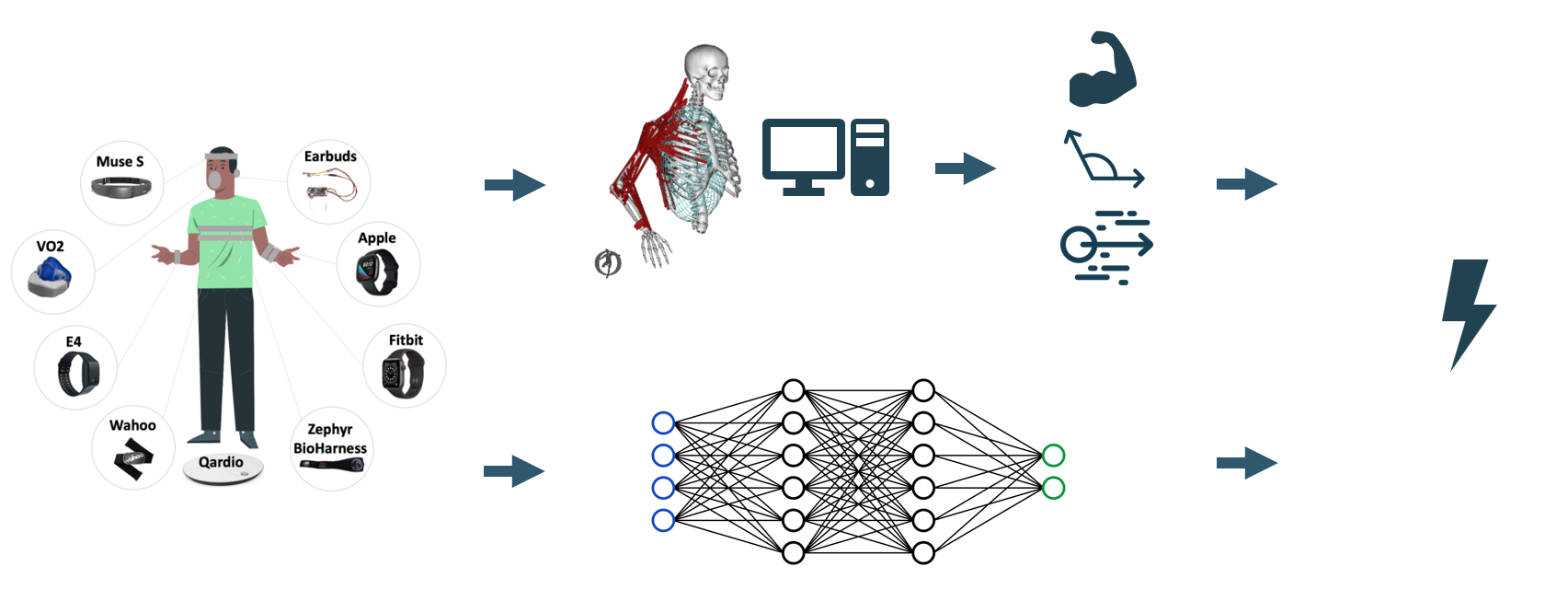
Ilias Masmoudi, M. Sc.
Department Elektrotechnik-Elektronik-Informationstechnik (EEI)
Lehrstuhl für Autonome Systeme und Mechatronik
- Email: ilias.masmoudi@fau.de